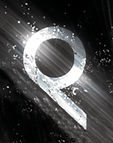

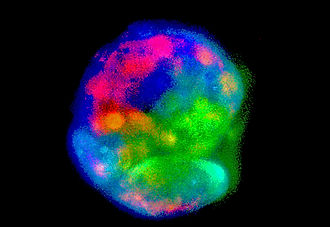
Intelligent Learning, Pattern Recognition and Bioinformatics
We have over 20 years of experience in the areas of pattern recognition, intelligent system design and algorithm development.
Our specialised computational facilities allow us to extract thousands of features from your multivariate data or images, whether single modality, multi-modality, correlative, or spectral.
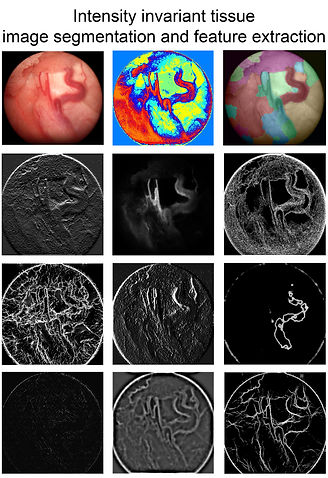
These features may be expert based or can be derived intrinsically from automatic deep learning based on your data set. These features maybe used for quantification, characterisation, classification or further image processing such as segmentation.

Using a variety of sophisticated pursuit and data projection methods, we can search complex multivariate spaces for evidence to support your hypothesis, and produce subspaces that reveal otherwise hidden structure, allowing you to easily visualise and validate the result. These may be trends, correlations, clustering, subpopulations, discriminations or any hypothesis you wish to explore for, followed by a full statistical analysis, testing and validation.
IPS cells were differentiated uses a variety of growth factors, the resulting differentiated cells formed host of subpopulations

Whether you wish to develop a specific robust detection or characterisation systems employing large multiple data sets and multiple groups or if you data is quite limited and difficult to obtain, we can build an optimised and cross validated system for you.
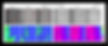
Quantitative is working in several commercial biomedical areas developing diagnostic tools.
We have a great team of software engineers able to provide you with a host of rapidly developed algorithms, toolboxes or commercial software.
Small Data Expertise....Getting Deep without Big Data
Brute force deep learning neural networks and associated tools have become completely affordable and almost common place, advertising push button convenience across multiple areas of research, engineering and finance. This pattern recognition approach typically relies on a very large number of observations or examples as it attempts to learn or extract both relevant and informative features from presented examples whilst subsequent neural layers try to determine how to best utilize these features to best predict or classify.
Such deep learning systems require little or no prior expertise and are able to perform well when given hundreds of thousands or millions of examples. However the very naïve nature of this process and the vast space of possibilities through which the network needs to search in order to find optimal solutions means they are largely inappropriate for most real world problems where the number of available observations is limited to hundreds or thousands of data points. Often the cost of obtaining more data is prohibitively high, and limiting the size of the training set for these systems can often result in the notorious problem of over fitting the data such that the solutions fail to work on new data, the generalization problem.
Our approach is quite different and embodies a library of expert inspired and cultivated feature algorithms which have embodied the knowledge of 20 years experience of image analysis methods, robotic machine vision, brain computer interface and life science microscopy in areas of embryology, cellular assessment and tissue pathology where we have created features derived across multiple studies and imaging modalities. This has progressively developed into a feature extraction algorithm library of a size greater than over a million available features per image channel and tens of millions for hyperspectral images.
With the ability to generate this vast and potentially highly informative feature set from a single image we avoid completely the necessity of the first layer of a deep learning system and the enormous amount of data required to create it. Then we employ an array of supervised, unsupervised, statistical, risk assessment and regularization tests to develop robust models and clusters of expert classifiers using only the optimal sets of these features.
This approach provides the very best of both prior knowledge and expert transfer learning, with robust classifier design. This approach has proven to be an excellent and reliable approach across many translational fields that rely upon robust pattern recognition of highly variable biological systems, prediction and assessment. We then work with the expert to further assess and understand particular observations or subgroups that do not fit into the model and use this to improve the sophistication of our classifiers, intelligence that would require perhaps tens or hundreds of thousands of examples to manifest in a deep learning system.
Our approach has produced significant outcomes based on both white light reflectance, phase and hyperspectral imaging technology, sensitive to both structural and biochemical factors, leading to significant progress in robust classification and prediction in the areas of embryology both human and Bovine, diseases such as motor neuron, cancer, inflammatory and genetic mutations, and altered cellular metabolism, differentiation and subpopulation discovery.
Dr. Martin.E.Gosnell B.E. (hons) PhD.
“For every dataset with one billion entries, there are 1,000 datasets with one million entries, and 1,000,000 datasets with only one thousand entries. So once the low-hanging fruit has been exhausted, the only possible way to move forward will be to climb the tree and build systems which can work with less and less data.”